-
-
Notifications
You must be signed in to change notification settings - Fork 3.5k
New issue
Have a question about this project? Sign up for a free GitHub account to open an issue and contact its maintainers and the community.
By clicking “Sign up for GitHub”, you agree to our terms of service and privacy statement. We’ll occasionally send you account related emails.
Already on GitHub? Sign in to your account
Resume training from official yolov3 weights #2
Comments
The loss is down, so I wounder whether the definition of loss lead to this problem? |
same issue, i change cls to 2(bg and person), it looks like cls confidence have sth wrong in training |
@lianuo @Ricardozzf I've not tried to continue training from the official yolov3 weights. It probably won't pick up smoothly where Joseph Redmon and company left off for a number of reasons, such as the optimizer starting with no knowledge of the previous optimizer's momentum and LR. There are also a few primary differences between my training and the official darknet training:
lcls = nM * CrossEntropyLoss(pred_cls[mask], torch.argmax(tcls, 1))
# lcls = nM * BCEWithLogitsLoss2(pred_cls[mask], tcls.float()) @lianuo how many epochs did you train this way? If you make the switch the BCE does this help? @Ricardozzf your results don't look good. Are you training from scratch or resuming training from yolov3 weights like @lianuo? If you suspect class confidence has a problem it must be because I've swapped CE for BCE. You can switch BCE back on by switching the commented lines above. But also note that if you are training from scratch you need significant number of epochs before things start looking good. In my training I see about 0.50 mAP on COCO2014 validate set after 40 epochs (3 days of training on a 1080 Ti). |
@glenn-jocher Thank you for reply! It is strange , that when I run test.py with this trained weight , I can still have high sore,see the screamshot: Is this because of the method of evaluate mAP? |
@glenn-jocher have you use the weights you trained (0.50 mAP on COCO2014) to test a image? |
@Ricardozzf thanks for you information.I am not alone ,haha. |
@glenn-jocher thanks for your reply I hope the information is useful to us. |
@lianuo @Ricardozzf thats a good question, I will compare my + Sample [4998/5024] AP: 0.7528 (0.4926)
+ Sample [4999/5024] AP: 0.8333 (0.4927)
+ Sample [5000/5024] AP: 0.5543 (0.4927)
Mean Average Precision: 0.4927 If I then use the epoch 37 checkpoint I'm wondering if I caused this by switching from BCE to CE. In xView when I used this code I had to increase my This is a bit apples and oranges comparison though. The official weights are at 160 epochs and my I don't understand why test.py is producing such a high mAP though, especially since it uses a very low
Any ideas are appreciated as well! |
@lianuo @Ricardozzf the overly-high mAPs you were seeing before should be partly fixed in the latest commits, which fixed mAP calculations (see issue #7). The official weights now produce .57 mAP, but the trained weights that before gave me 0.50 mAP now return about 0.13 mAP, much more in-line with the poor boxes you see in your images. I still don't understand the actual cause of the poor training results however. |
@glenn-jocher Thank you for reply~ |
@glenn-jocher the loss is still decrease when training ,do you think the loss function need to modify? |
No warm-up process found for SGD. According to the paper of YOLO9000 and the official code, we need to warm-up the first 1000 iterations to make it better converge: |
@glenn-jocher The usage of CrossEntropyLoss might be incorrect. The input shape is (nB, nA, nG, nG, nC), but the pytorch-doc suggests it to be (nB, nC, ...). (See Line 115 in 9514e74
Besides, the torch.argmax(tcls, 1) fetches C from dim=1, but the shape of tcls is actually (nB, nA, nG, nG, nC). Maybe we need to permutate the dims so that C is at dim=1 . |
@xyutao I looked into the CELoss function, I think this part is ok. When I start training and debug this spot, the dimensions look good (assuming tcls.shape
Out[2]: torch.Size([12, 3, 13, 13, 80])
tcls = tcls[mask]
tcls.shape
Out[3]: torch.Size([47, 80])
lcls = nM * CrossEntropyLoss(pred_cls[mask], torch.argmax(tcls, 1))
Out[4]: tensor(206.37325, grad_fn=<MulBackward1>)
pred_cls[mask].shape
Out[5]: torch.Size([47, 80])
torch.argmax(tcls, 1).shape
Out[6]: torch.Size([47]) I linked to your comment on the SGD warmup however, this is a good catch! Issue #4 is open on this. By the first 1000 iterations do you mean the first 1000 batches? |
@glenn-jocher Yeah, the first 1000 batches of batch_size=64. |
please help,i have the same error, |
@lianuo Hi, just wondering how you loaded a pre-trained weights. Did you add this line of code in train.py?
|
@lianuo I found out from detect.py that you add this line:
But, now, I'm getting a different error from datasets.py: Have you encountered this problem; if yes, how do you deal with it? |
@jaelim you resume training from a trained model (i.e. latest.pt) by setting Lines 50 to 53 in 68de92f
If you are seeing the error you mentioned it is because you failed to define a proper path to an image, or image folder in detect.py line 14 (no images are loaded). Make sure there are only image files in the path if you specify a path. Also please do not ask questions unrelated to the main issue title in this thread. Line 14 in 68de92f
|
@xyutao I've switched from Adam to SGD with burn-in (which exponentially ramps up the learning rate from 0 to 0.001 over the first 1000 iterations) in commit a722601: Lines 115 to 120 in a722601
![]() Unfortunately this caused Lines 121 to 131 in a722601
If I plot both of these in MATLAB it looks like the lack of a ceiling on the original code is causing the divergence problem. It may be that the original width/height equations are incorrect. Does anyone know where to find the original >> x=linspace(-3,3);
>> y1 = exp(x);
>> y2 = ((logsig(x) * 2).^2);
>> fig; plot(x,y1,'.-'); plot(x,y2,'.-'); h=gca; h.YLim=[0,5]; legend('original','updated'); xyzlabel('network output','anchor width multiple'); fcnfontsize(14) |
@lianuo @Ricardozzf @xyutao @CF2220160244 @jaelim I have good news. A significant bug in the loss function was found today in issue #12, namely a problem I fixed this in commit cf9b4cf, and after the change observed that SGD with burn-in now converges with the original YOLO width/height calculations, so I placed those back in in commit 5d402ad. Update: Sorry guys I think I might have spoken too soon. The changes help, but resuming training from yolov3.pt still causes P and R to drop from initially high values to lower values after ~50 batches. I think we are getting closer to the source of the problem however, which I feel is in the model loss term somewhere. TODO: I also need to ignore non-best anchors with > 0.50 iou to match yolov3. |
@sporterman Sorry, i haven't trained my own dataset. |
@glenn-jocher Thanks for your reply and great work. I have varied |
@deeppower yes the performance is still not as good for training as darknet unfortunately. I tried a few epochs of multi_scale training after epoch 80 and this did not seem to help. I've tried to align everything as closely as possible to darknet, so for example if you resume training from the official yolov3.pt weights the P and R values are very steady (though still dropping slightly over time). This makes me think the loss function is correct, or at least very close to the original darknet loss function. Inference works well, so the problem can not be there, it must be in the training-only code, which could be optimizer, LR scheduler, loss function, target building functions, IOU function, augmentation function... |
@nirbenz @okanlv @deeppower @okanlv @xiao1228 Good news I think. I thought about the problem a bit and decided that the loss terms needed rebalancing. In my last plot you can see Classification is consuming the great majority of the loss, which means that it is being optimised at the expense of all the other losses. Ideally the 6 losses would be roughly equal in magnitude so that they are all optimised with equal priority. So I made a commit that multiplied Objectness loss by 10, and divided Classification loss by 10: Lines 166 to 176 in e04bb75
I ran this for most of the day on GCP, and after about 10 epochs I overlaid the 3 different trainings I'd done. This new approach seems vastly better, in particular at increasing Recall compared to before. I thought this was exciting enough to post the news right away, I'll have to train for another week to get to 70+ epochs and see the true effect. I'm wondering if there isn't a better way to more automatically balance these 6 equally important loss terms. They seem roughly equal now after 10 epochs, but maybe theres a way to update the balancing terms every epoch with the previous epochs gains. Any ideas? UPDATE 1: mAP is 0.43 ( |
@deeppower Yes, objectness loss is higher than before because I multiplied it by 10x now. I'm trying to balance the loss terms so they contribute equally to the gradient, or else the largest loss terms will get optimized at the expense of the smaller loss terms. It appears to be working, though my loss term multiples are rather arbitrary unfortunately. Ideally we want to take this a step further, and better equalize not just the loss terms, but the target distributions to something like zero mean and unity variance, which helps regression networks at least (not sure about object detection). Any experiments you can run on your own would help significantly, I'm just one man with one GPU here, so I can only try a finite sets of things to improve the results. |
@okanlv I have a question for you. Now that I've defaulted to start training from darknet53.conv.74, would it make sense to freeze those layers for a bit of time before allowing them to change? I was thinking I could freeze them for the first epoch perhaps, which would be 7328 batches, or half epoch at least. The first 1000 batches are burn in. I feel like it would make sense to do this since the randomly initiated layers might converge must faster without the darknet53.conv.74 layers changing underneath them. |
@glenn-jocher In the darknet repo, all layers are trained together after yolov3 is initialized with darknet53.conv.74 weights. In this paper, the authors have showed that updating the parameters of all the layers increases the performance compared to updating the parameters of only the top layers (related to fragile coadaptation of the layers, mentioned in the paper). That being said, your method might also work, because there are a few differences between your approach and the experiments in the paper. If you train yolov3 with your approach, could you share the loss graphs including your approach and the current method? It could be beneficial for further experiments. |
@nirbenz @okanlv @deeppower @okanlv @xiao1228 I've started running studies to improve the COCO map when training from darknet53.conv.74. I started with the #2 (comment) model that gets 0.46 mAP at epoch 35. The primary breakthrough there was simply rebalancing the loss terms, multiplying All tests below are only run for the first epoch. Freezing the darknet53 layers (just for the first epoch) showed slightly positive results. It seems further rebalancing the loss terms has the biggest effect. In most ML regression problems the inputs and targets are always recalibrated to zero mean and unity variance, yolov3 does this for the inputs via Any other experiments you guys want let me know. I'll keep populating this comment as my results come in over the next week.
This is my selected configuration, + |
Thanks for your improvement of this YOLOv3 implementation.
I have just test the training ,got some problem .
I follow these steps.
3.Got the following logs ,the precision is down fast from 0.5->0.1. but recall is up to 0.35.
see Screenshot here
4.I save the weight with precision0.2, and run the detect.py
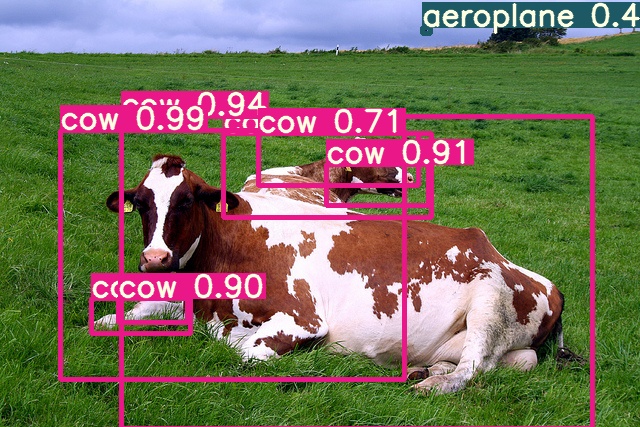
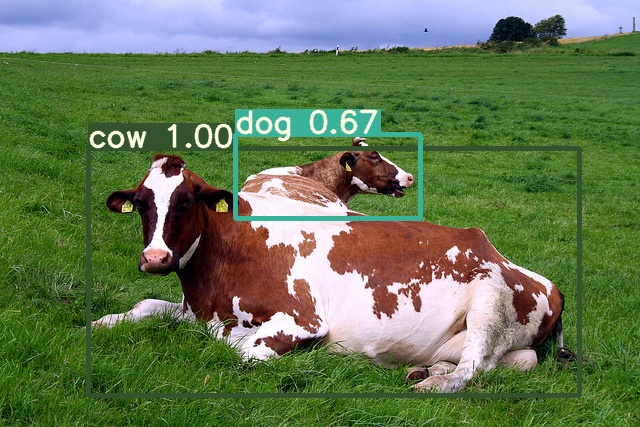
the result like this ,
if I do not train,the orginal wight can get this result:
I do not know whether I used wrong parameters or something else, lead to generation of many bbox .
could you give me some suggestion?
Thank you~
The text was updated successfully, but these errors were encountered: